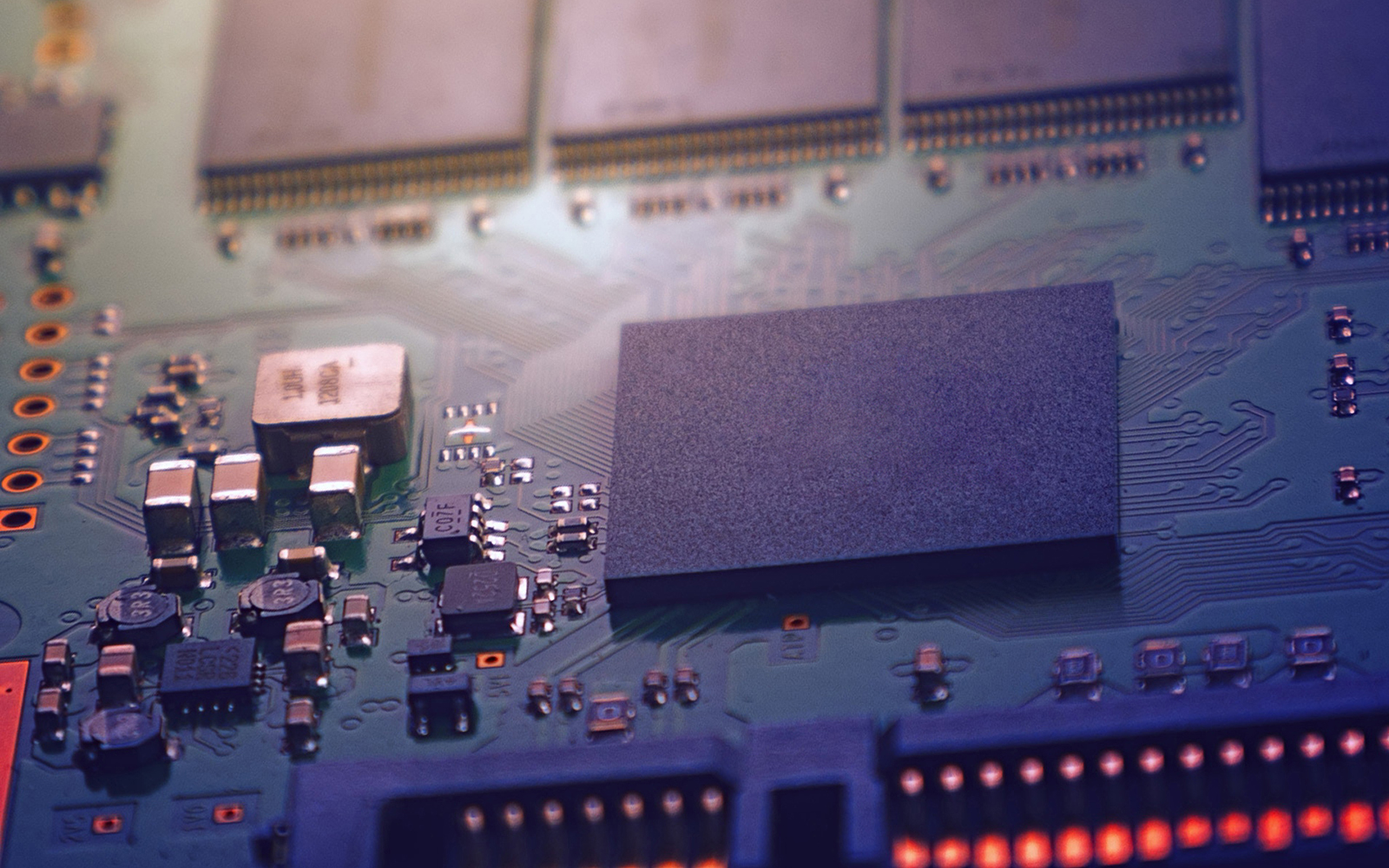
Written by Tony King-Smith / Posted at 11/27/20
Exploiting parallelism in NN workloads to realize scalable, high performance NN acceleration hardware
Many automotive system designers, when considering suitable hardware platforms for executing high performance NNs (Neural Networks) frequently determine the total compute power by simply adding up each NN’s requirements – the total defines the capabilities of the NN accelerator needed. Or does it?
The reality is almost all automotive NN applications comprise a series of smaller NN workloads. By considering the many forms of parallelism inherent in automotive NN inference, a far more flexible approach, using multiple NN acceleration engines, can deliver superior results with far greater
scalability, cost effectiveness and power efficiency...