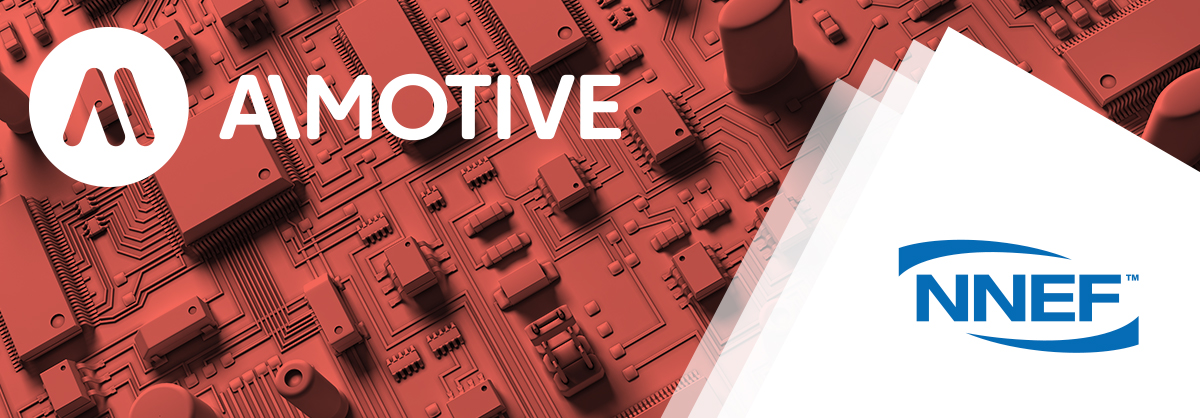
Written by Viktor Gyenes / Posted at 8/30/18
How AImotive Uses NNEF
As one of the initiators and Spec Editor of NNEF™, AImotive is an early adopter of the framework. Working on NNEF with our partners in the supportive framework of the Khronos Group has been an enlightening experience. As a result, our aiWare hardware IP is one of the first deep learning hardware platforms to offer NNEF support through a comprehensive SDK. Furthermore, our internal and external work processes also rely on the standard.
Fragmentation: Hindrance, not catalyst – At an early stage of our research into autonomous vehicle artificial intelligence, we realized that the huge amount of choices in both deep learning frameworks and hardware platforms would lead to difficulties. As artificial intelligence research gains momentum we have used three frameworks over the past few years. Our first networks were trained in the Caffe framework, later we switched to TensorFlow, and currently, PyTorch is gaining importance.
In effect, a lot of our earlier work would be obsolete without NNEF. Our legacy neural networks would only be accessible through time and resource consuming conversions. To counter this, we have begun to create an archive of NNEF descriptor files for all the neural networks AImotive has created. Our current research is already stored in NNEF descriptor files, regardless of which deep learning framework was used. This ensures that despite any changes in the tools we use, our earlier work remains readily accessible when needed.

Following through with our use of NNEF in our development, we also use the standard to export final networks for use on the GPUs in our prototype cars on the road, and any demo networks provided with our aiWare FPGA Evaluation Systems. Offering an SDK with full NNEF support for all future aiWare chip-based platforms allows those using NNEF and relying on our hardware architecture and SDK to cut back on the overhead of changing to our neural network hardware acceleration platform. In turn, this guarantees that their software solutions will work seamlessly when they make the switch to aiWare. This also means that when we switch GPUs for aiWare chips in our test cars, our software will continue to work in a stable manner.
Providing the freedom to create – As providers of software technologies that heavily rely on artificial intelligence, the hardware platforms our partners choose have always affected the format in which we provided different software versions. For example, if our partner uses an inference engine based on the Caffe format, we will have to use the Caffe framework for training. Moving forward we will increasingly rely on NNEF to share our technology with our partners. This will ensure fewer limitations to their choice of inference engine. As a result, they can follow their most effective development paths, as can AImotive, to ensure the best possible solutions and platforms are adopted.
NNEF offers us the freedom to create software solutions that are unconnected to the deep learning frameworks used to train them and the hardware platforms used to compute them. This drastically increases our possibilities in choosing the tools to develop our technology.
For AImotive, NNEF is already living up to expectations and tackling several problems caused by the fragmentation of artificial intelligence deployment. We hope the standard will continue to grow, and be adopted by an increasing number of industry players to strengthen cooperation and accelerate the adoption of AI-based solutions.